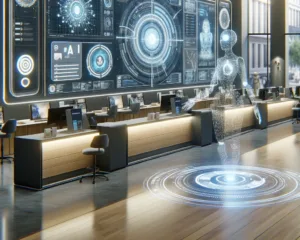
Exploring the journey from legacy banking systems to AI integration, focusing on practical strategies for data governance, targeted AI applications, and maintaining a balance between machine efficiency and human oversight to truly revolutionize digital banking.
Artificial intelligence (AI) is dominating digital banking headlines with grand promises to revolutionize everything from customer service chatbots to anti-money laundering detection. However, the path from hype to reality is often paved with complexities, leaving many institutions struggling to bridge the gap between legacy systems and cutting-edge AI capabilities. While core modernization roadmaps now routinely integrate AI components, tangible progress remains sluggish, largely due to the practical challenges of embedding intelligent algorithms within systems never designed for machine learning models.
Getting Governance Fundamentals Right
Establishing proper data governance marks the critical first phase of any AI program within the banking sector. Core systems house the most transactional information, yet decades of mergers, acquisitions, and disjointed modernization cycles have created convoluted environments. To navigate this complexity, assembling cross-functional Data Councils brings together leaders from IT, security, compliance, lending, and more, aligning on policy frameworks. This foundational step provides structure for capturing, protecting, and managing information flows that fuel AI engines, while also demonstrating governance to earn the green light from risk functions and regulators.
Start Small, Build Momentum
The most successful institutions take an agile approach to proving AI capabilities before attempting to tackle massive challenges all at once. Targeting contained opportunities within specific products allows bankers to walk before running. For example, building a basic propensity model to predict which customers may convert to new lending products requires much less lift than re-architecting an end-to-end Small Business loan lifecycle powered by machine learning. However, getting a few quick wins under their belt gives confidence for bankers to expand AI across broader applications.
Cultivate Connective Tissue
Legacy platforms often communicate across dated protocols never designed for the data demands integral to AI. While modern architectures eventually replace aging infrastructure, connectivity improvements make machine learning more accessible in the interim. API layers essentially serve as translators, allowing legacy systems to share information flows with more analytics-optimized data lakes and warehouses. Though progressing core modernization remains imperative over the long-term, API integration accelerates AI ability today.
Start Identifying “Bright Spots”
Innovating with AI requires moving beyond thinking solely based on risk parameters. Forward-leaning bank strategists spot customer pain points ripe for AI-enabled enhancement, particularly around digital experiences. For instance, chatbot concierges with more emotionally intelligent responses can handle common inquiries to offload contact center volume. Similarly, personalized insights revealed through data analysis may uncover new cross-sell options. Starting with customer-centric perspectives guides AI integration toward better business outcomes beyond purely operational gains.
The Human Touch
While AI delivers data-fueled recommendations, human experts add the judgment required for trustworthy outcomes. Combining machine precision with human nuance allows banks to amplify expertise rather than outright replace jobs with robots. Workflow integrations that loop humans-in-the-loop ensure quality control like validating fraud alerts before automatically declining transactions. Ethical oversight also helps algorithms avoid bias tendencies that emerge within AI absent governance. Partnering humans with machines checks AI’s thirst for data while keeping decisioning accountability firmly within human purview.
Make Haste, Slowly
AI implementation compresses years of accelerated technology adoption into highly compacted timeframes. Such unprecedented change intensifies transformation hurdles as bankers build capabilities in data, talent, and culture. Rather than rushing headfirst without proper pacing, savvy digital bankers instead commit to gradual progress fueled by patience. Slowing down to document institutional knowledge passed down verbally for decades futureproofs banks against existential skills gaps as older experts retire. Cross-pollinating data and technology talent through upskilling programs similarly prevents resource bottlenecks.
While competitive forces pressure rapid innovation, methodical transformations outperform reactive responses. Building sustainable foundations for optimizing data, spreading AI literacy companywide, and aligning to supervisory expectations steers banks beyond experimental phases into scaled production. AI holds massive promise for takeoff across banking, but pragmatic core bankers recognize achieving orbit depends first on completing the pre-flight checklist. Following deliberate steps for governance, integration, and responsible development manifests AI’s automation superpowers through a distinctly human touch.
Found this article interesting? Check out these three related reads for more.
- AI integration in legacy banking systems the agile advantage
- AI-driven core banking modernization Revolutionizing customer experience and operational efficiency
- Enhancing the digital customer journey in banking with AI, ML, and Blockchain
#AnalyticsInBanking #AgileBankingTransformation