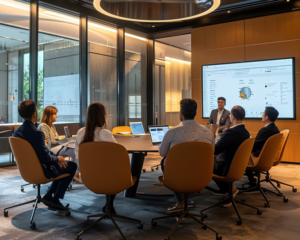
Highlighting strategies for combating AI bias in banking, ensuring fair and ethical AI integration through proactive auditing, responsible design, and continuous vigilance.
As banks increasingly leverage artificial intelligence, a major challenge that could derail progress is the issue of bias. Uncontrolled biased data, algorithms, and machine learning models will likely produce biased and unethical results. However, by implementing clear strategies, institutions can innovate with AI in a fair and responsible manner.
Recognizing sources of bias is the first step:
- Biased Data: Historical data used to train models may reflect societal prejudices
- Biased Algorithms: The way algorithms are programmed can directly or inadvertently privilege certain groups
- Biased Outputs: Even neutral models can develop discriminatory outputs over time
Proactive auditing of datasets, algorithms, and model outputs is required to identify bias.
Preventing bias through responsible AI design principles is key:
- Develop bias testing frameworks and model risk management policies
- Use training data representative of all demographics
- Build explainable AI models with transparent logic to evaluate fairness
- Enable human oversight to audit model behavior and override problematic outputs
- Automate frequent bias testing into the model development lifecycle
Where bias already exists, corrective measures are needed:
- Neutralize bias by reweighting data and editing algorithms to remove prejudice
- Rebuild models from the ground up using sanitized data and redesigned algorithms
- Analyze outputs to ensure skewed/discriminatory results are eliminated
Maintaining continual vigilance through proactive bias prevention principles like mitigation frameworks, diversified data, explainable models, human oversight, and automated bias testing is crucial. This ensures trustworthy and transparent AI innovation that treats all groups fairly.
In the AI era, prioritizing these fairness-ensuring strategies seals the deal for responsible adoption of AI in banking services for the ethical benefit of all customers.
Found this article interesting? Check out these three related reads for more.
- Mitigating bias in banking algorithms
- AI-driven core banking modernization Revolutionizing customer experience and operational efficiency
- AI-driven banking personalization Leveraging big data for banking insights
#AIFairness #BankingInnovationAI