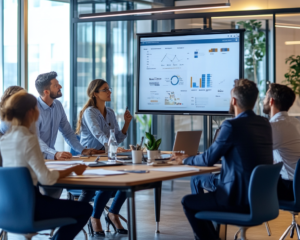
Exploring the pivotal role of big data and advanced analytics in transforming banking operations, enhancing risk management, and driving digital transformation in the financial sector.
Given the face of banking, Big Data, and Advanced Analytics that’s changing so fast, they’re no longer a fad but a given. Strategic use of data and machine learning is fast turning out to be a cornerstone in risk management, decision making, and overall business transformation as banks learn to negotiate the challenges of the new digital world. Now that we’ve talked it through, let’s review some of the most salient points and key take-aways that have come out of this research on the subject. So what does all this mean for the banker?
The New Era of Data: Traditional Meets Big Data
Banks have always dealt with data: customer transactions, credit history, market data. Big Data added a twist to this process. We are at that point where structured, conventional data is combined with oceans of unstructured, external data-from demographic insights to information churned out by social networks. This integration of data types enables more profound insights and more accurate predictions about customer behavior.
Access to conventional and Big Data sources should be possible simultaneously. In other words, banks can use this combined data source to create more sophisticated predictive models for better segmentation of their customers and to offer them only those financial products that match their needs.
Big Data’s 7V Model: An Extension of the 7V Model
Big Data often comes in what is known as the 3V model, which stands for Volume, Variety, and Velocity. Nowadays, big data has become a banking framework with the addition of four Vs: Value, Veracity, Variability, and Visibility.
- Volume: The actual amount of data gathered.
- Variety: The amount of diversity within the given data.
- Velocity: The rate at which data comes in and is forwarded.
- Value: Relates to the importance of the data in presenting useful insights.
- Veracity: Data correctness and verifiability.
- Variability: Inconsistency in data identified and handled effectively.
- Visibility: Making data visible by means of visualization.
Understanding and applying the 7V model, banks can optimize their usage of data by ensuring that not only large amounts of data are brought in but also meaningful and reliable data.
Big Data and Advanced Analytics-the Four Pillars in Banking
The European Banking Authority commented that the following four pillars will provide a way to use Big Data in banking:
- Data Management: Governance regarding quality and security for a variety of data sources.
- Technological Infrastructure: Proper data platforms with related processing capabilities.
- Organization and Governance: Clear roles and responsibilities, and flows of communication.
- Analytics Methodology: Development and adoption of advanced analytics techniques including machine learning.
Each of these pillars is important to a bank’s journey of digital transformation. Without strong data management and infrastructure, advanced analytics cannot achieve their full potential.
Machine Learning: The Driving Force Behind Advanced Analytics
Machine learning (ML) is pretty much catching up with the whole drastic change in banking operations. Starting from fraud detection to credit scoring, algorithms of ML are being applied to process huge amounts of data at incredible velocity and accuracy. Four major types of Machine Learning relevant in banking include:
- Supervised Learning: The algorithm learns on labelled data to predict the target variable, such as spam detection.
- Unsupervised Learning: Algorithms find patterns in unlabelled data, useful in customer segmentation.
- Reinforcement Learning: These algorithms learn through trial and error but find broad applications in automated systems, such as robots.
- Deep Learning: This would be part of Machine Learning that involves a neural network consisting of many layers. It finds applications in the field of image recognition and NLP.
ML is not just a hype, but powerful, as it has the potential to improve the processes of decision-making, mitigate risks, and bring efficiency in various banking functions.
Risk Management in the Big Data Era
One of the major areas where Big Data and ML have their highest impact is in risk management. Banks can do a better assessment of credit risk, monitor portfolio quality, and even sometimes forecast financial crime through large data analysis. Techniques of ML like decision trees and regression analysis find broad applications in developing internal ratings-based models, using advanced techniques to accommodate regulatory requirements.
We recently helped a Regional US Bank to deploy Exabeam and a new draft operating model with Fraud, Compliance, Risk and Financial Crimes Departments. Exabeam uses AI and machine learning to continuously evolve its security analytics platform. Exabeam is primarily known for its User and Entity Behavior Analytics.
Big Data and ML are integral in risk management, allowing for improved decision-making on banking risks more precisely and with urgency.
Key Challenges and Considerations
Admittedly, benefits cannot be denied here neither can some of the challenges that explain overfitting, explainability of models, and possible biases among only a few of the major problems in need of solution. Normally, when an algorithm is overly complex, this fails to generalize well and results in overfitting. Explainability tends to undermine the stakeholder trust in ML models, while bias is one driver toward unfair or discriminative outcomes that cause negative challenges.
All these challenges regarding transparency, equity, and robustness of the ML models need to be known to the banks to make sure that validity is ensured. They would require continuous monitoring and validation in order to keep up the integrity of the systems.
Ushering in and Embracing the Future of Banking
Big Data and Advanced Analytics are here to stay, and the deeper we go into the digital era, the bigger their role in banking. That would mean the message to bankers is: adapt or die. It is with these technologies that banks will manage not only to keep pace but even beat the pace of innovation towards an efficient, safe, and customer-oriented financial system to the benefit of all in the end.
After all, this is not about keeping pace with technology but harnessing the power of technology to bring transformation and create new opportunities in banking. Thus, while on this journey of digital transformation, he or she would always find it worth remembering that the future of banking rests in the core of data, and that the key to unleashing it lies in its usage.
Found this article interesting? Check out these three related reads for more.
- Mitigating bias in banking algorithms
- Core banking transformation with embedded analytics
- Data analytics for competitive advantage in banking – From legacy to leadership
#CoreBankingTransformation #BankingKeyPoints